Research
Statistical Inference and Machine Learning
My core research is concentrated at the intersection of statistics and machine learning. Traditional statistical methods often produce models that are intuitive and under mild regularity conditions, can easily produce standard forms of inference such as confidence intervals and hypothesis tests. However, they often require the user to designate a particular form or structure on the underlying relationships between variables and when incorrectly specified, the results may be suspect. Statistical and machine learning methods take the opposite approach, instead adapting to the data at hand. When sufficient data is available, they will often dominate more structured models in terms of predictive accuracy. Unfortunately, this inherent flexibility also means that most learning algorithms are notoriously difficult to formally analyze, often resulting in "black-box" models in which understanding how predictions were generated or what relationships might exist in the data is largely hidden.
The bulk of my work revolves around trying to bridge these ideas. Usually this means attempting to enforce some structure on learning algorithms that will allow for basic distributional results (which then allows for inference) without destroying the key components of the algorithms that produce accurate predictions. You can see some of our results for random forests here and here.
One area of focused application is the ebird project hosted at the Lab of Ornithology at Cornell University. This citizen science project relies on individuals to submit checklists of bird observations which are then collected, aggregated, and used to (among many other things) produce occurrence maps such as that shown below. Our work utilizes this data and allows researchers to formally investigate why a particular species might be more likely to be in one area or another as opposed to only predicting the probability of being in a particular area.
The bulk of my work revolves around trying to bridge these ideas. Usually this means attempting to enforce some structure on learning algorithms that will allow for basic distributional results (which then allows for inference) without destroying the key components of the algorithms that produce accurate predictions. You can see some of our results for random forests here and here.
One area of focused application is the ebird project hosted at the Lab of Ornithology at Cornell University. This citizen science project relies on individuals to submit checklists of bird observations which are then collected, aggregated, and used to (among many other things) produce occurrence maps such as that shown below. Our work utilizes this data and allows researchers to formally investigate why a particular species might be more likely to be in one area or another as opposed to only predicting the probability of being in a particular area.
An ebird occurrence map for Indigo Buntings produced by the Lab of Ornithology at Cornell University. http://ebird.org/content/ebird/occurrence/indigo-bunting/
Variable Selection and Non-parametric Inference
Another broad area of growing interest to me is variable (feature) selection. As described above, for simple explicit models, such measures are typically natural and widely available. However, when such models fit poorly, we might not trust the results. Learning methods on the other hand generally fit well but seldom produce any analogous reliable measures and those ad hoc measures that do exist can usually be easily tricked by things as simple as mild correlation between predictors. I'm interested in developing robust methods to identify important subgroups of predictors, especially in big data (large n) and high-dimensional (p > n) settings.
Forensic Science
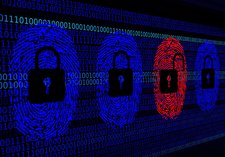
I had the opportunity to spend the 2015-2016 academic year at SAMSI working in the program on statistics and forensic science. A 2009 report by the National Research Council found that outside of DNA analysis, nearly all forensic analysis techniques lack significant scientific and statistical backing. While at SAMSI, I worked alongside leading researchers from across the world to investigate these issues, paying particular attention to issues related to confirmation or contextual bias and also in developing quality metrics for latent pattern evidence based on new image decomposition techniques. You can find a preprint of some of our methodological work here; work related to the extension of these methods to quality metrics is currently in progress.
I am also currently working with the Houston Forensic Science Center to develop blind quality control procedures for the analysis of forensic evidence and to explore various sources of bias and uncertainty in the reporting of results. You can find some recent Op-Eds written with visiting SAMSI colleagues here and here as well as related write-ups by the Innocence Project here and here.
I am also currently working with the Houston Forensic Science Center to develop blind quality control procedures for the analysis of forensic evidence and to explore various sources of bias and uncertainty in the reporting of results. You can find some recent Op-Eds written with visiting SAMSI colleagues here and here as well as related write-ups by the Innocence Project here and here.
Law and Juror Perceptions
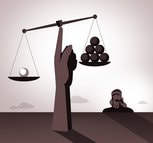
In addition to working to strengthen the manner in which forensic evidence in analyzed, I'm also very interested in the way(s) in which that evidence is reported in criminal trials and how jurors perceive the strength of such evidence. Of particular importance is determining how the typical juror might weigh the strength of a particular piece of forensic evidence against his or her prior beliefs, how judges presiding over a trial might best instruct juries to interpret results and conclusions with minimal prejudice, and how jurors adjust for effects of bias and uncertainty (both reported and unreported).
Other Applications
In addition to those application areas mentioned above (ecology, forensic science, and law) I'm also interested in biomedical applications, predictive policing (crime prediction), and sports analytics. You can find a recent interesting investigation into the role of metabolism in DNA methylation here.
Media
- A recent interview with Giles Hooker discussing some of our work together
- Some coverage by USC of the Data Science Innovation Lab that I took part in during the summer of 2016
- An Op-Ed in the Houston Chronicle I co-authored with Maria Cuellar (CMU), Cliff Spiegelman (Texas A&M), and Bill Thompson (UC Irvine) on scientific progress made by the Houston Forensic Science Center. Subsequent coverage by the Innocence Project.
- An Op-Ed in the Pittsburgh Post-Gazette I authored with Maria Cuellar (CMU), Cliff Spiegelman (Texas A&M), and Bill Thompson (UC Irvine) on problematic scientific issues in forensic science, with respect in particular to the Steven Avery case and the Netflix docuseries "Making a Murderer". Subsequent coverage by the Innocence Project and Bustle.
- A short profile and interview during my time at SAMSI